
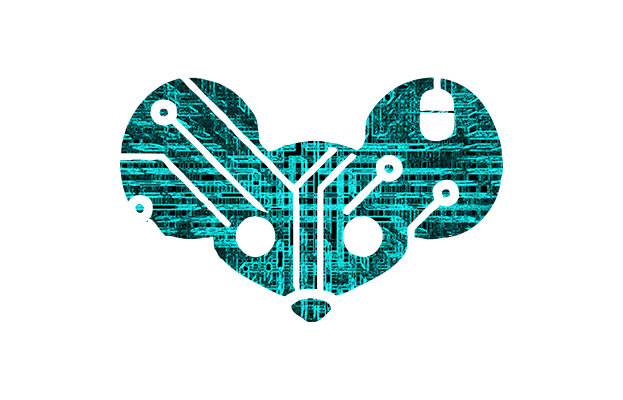
One of my big beefs with ML/AL is that these tools can be used to wrap bad ideas in what I will call “Machine legitimacy”. Which is another way of saying that there are many cases where these models are built up around a bunch of unrealistic assumptions, or trained on data that is not actually generalizable to the applied situation but will still spit out a value. That value becomes the truth because it came from some automated process. People cant critically interrogate it because the bad assumptions are hidden behind automation.
The day to day reality for me at least is that the new hyped up llms are largely useless for work and in some cases actually detriments. Some people at work use them a lot, but the heavy users tend to be people who were bad at their jobs, or at least bad at the communication aspect of their jobs. They were bad at communicating before and now, with the help of chat gpt, they are still bad at communicating, except they have gotten weirdly obstinate about their crappy work output.
Other folks I know have tried to use them to learn new things but gave up on them when they kept getting corrected by subject matter experts.
I played around with them for code generation but did not find it any faster than just writing and debugging my own code.